Now and again — actually almost every day — we get a question from the community or from our commercial users about performance.
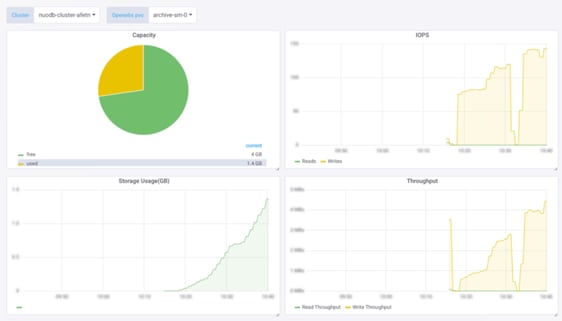
IOPS SCREENS FROM MAYAONLINE
Our typical response is “mileage may vary.” We also occasionally mention that there are several tunables, then talk to us for help. By the way , we love to discuss performance requirements as it shows off how CAS is different, and we also get to learn much more about how OpenEBS is being used.
This blog post shares some high-level points on tuning, and then more detailed information that can be put into practice by users.
First, it is worth noting that Container Attached Storage — where the workload is married to the storage controller — is by definition per workload storage. So, when you compare OpenEBS to CEPH, for example, or some other pre-Kubernetes antediluvian scale-out storage system, you risk apples to oranges comparisons. While CAS will be constrained by the environment itself and how many resources OpenEBS utilizes from Kubernetes, it will not be directly impacted by additional workloads. Those workloads are essentially an island unto themselves. By comparison, a system that checks with a metadata store to determine where to write and where to read the data and stripes data across nodes, for example, will have far greater performance impacts as more workloads are added.
Secondly, as I mentioned on twitter the other day, sometimes the performance you are looking to optimize should be human as opposed to workload. With OpenEBS running natively in Kubernetes, you can spin up storage in seconds on a per-workload basis and manage it in a very familiar Kubernetes way. No external distributed system run on a foundation other than Kubernetes can be as simple to run and scale. You’ll need a team of experts to run that external distributed storage system — experts that arguably should be applied to running the distributed system you are betting upon — Kubernetes itself. You’ll inevitably find that it takes weeks to understand any external distributed state store and years to understand how it will behave in the real world. By comparison, if you know how to run Kubernetes, you already know how to run OpenEBS. So, if you compare the human factor of storage performance — the humans and their ability to understand and run the storage software itself — there is no real comparison between OpenEBS and any other storage. (yes , I see my fellow members of the CAS category and I know what having a kernel module means for ongoing operations :)).
Alright, enough with the high-altitude view! If you are still reading, I assume you want to know the details about tuning OpenEBS.
The good news is that you can tune cStor — which is our more enterprise-focused storage engine released in 2018 — based on the characteristics of your workload. We are working on adding these configurations directly to your storage classes as part of the forthcoming 0.9 release (we are on 0.8.1 currently) to support the infrastructure as code pattern.
Here are the two main tuning that can help improve performance:
- Scheduling the application and associated cStor storage pods
- Tuning the cStor volume based on the application IO pattern
Scheduling
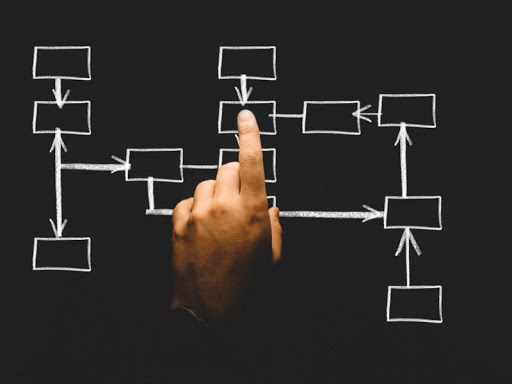
Scheduling the Application and Associated cStor Storage Pods
cStor is the OpenEBS storage engine written in C. It is Kubernetes-native and follows the same constructs as other application pods, where affinity and anti-affinity rules can be specified to localize the access to the data and to distribute the storage pods to be resilient against node and zone failures. cStor uses synchronous replication behind the scenes to keep copies of your data, so the network latency between the cStor target and its replicas will have a performance impact that needs to be accounted for when designing the system for resiliency. Note that quorum policies are configurable, so you can specify if you want cStor to acknowledge writes when received or, more typically, when received and then confirmed by one or two replicas. Perhaps not surprisingly, performance is best when the application, the cStor target, and its replicas are co-located or connected via a fast network.
Tuning the cStor Volume
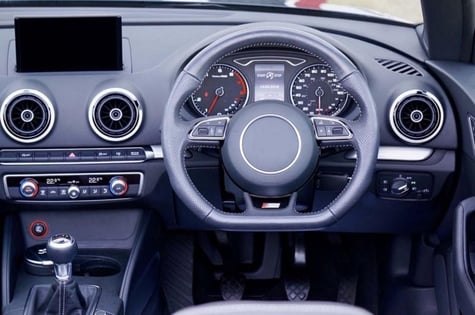
The two main tunings are LU, or “lun worker,” and queue depth, which is simply the depth of the queue used in IO for the OpenEBS target. By default, when you turn up OpenEBS and use the default configurations, OpenEBS runs in a lightweight manner. However, performance improves as you add more CPU to add more workers to LU and QD.
It is possible to modify the default settings via `kubectl exec` and if you would like to try these out, feel free to contact us through the OpenEBS Slack community. With 0.9, the tunables will be available as with other storage policies that OpenEBS currently supports via storage class and persistent volume claim annotations.
You can read more about these storage policies both for cStor and for the earlier Jiva based storage engine on our docs here:
OpenEBS Storage Policies ·docs.openebs.io
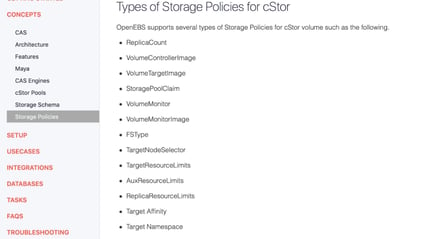
Keep in mind that optimizing performance based on environmental and workload characteristics can be fairly complex. For example, performance often depends upon whether your workload is sequential or random and of course whether it is largely read or write. Many transactional DBs are sequential write heavy, whereas analytic workloads are often sequential read heavy and logging is typically more random writes. Also, the block sizes matter as does the characteristics of the underlying storage device (disks vs. SSDs for example). In the case of sequential workloads on cStor, your write performance may actually degrade with additional workers. In that case, you would want to decrease the workers to only one thread.
Again , remember that if you are on this path we are happy to help you and learn from your experience.
We have a variety of automated routines coming to assist in performance testing in OpenEBS 0.9 and later releases, and in MayaOnline. There is a vast amount that can be done by closing the loop between configuration and actual performance as monitored — especially when combined with an active testing or chaos engineering solution such as Litmus. While there is some performance testing in Litmus, we and others in the community are adding workload tests such as TPS or transaction tests for various DBs that are frequently run on OpenEBS (thank you, Cassandra, NuoDB, MongoDB and other friends). We are also working on additional closed-loop optimization to build into OpenEBS operators and make visible via MayaOnline. As always, we welcome your feedback and suggestions!
So, when might you want to make changes to tune your storage for performance? Typically, we see requests for these changes in environments in which storage is the primary performance bottleneck. As an example, we have a large IoT provider that is using cStor in thousands of remote nodes. This user knows the hardware underlying those nodes and their likely workloads. They felt that it was reasonable to maximize the resource usage of OpenEBS in order to expedite local on node performance of a random heavy workload.
On the other hand, if you are most interested in saving money and are running the workloads in clouds where CPU usage is relatively expensive, then you may prefer to run OpenEBS in a lightweight manner with as few workers as possible. Keep in mind that a common use case is running OpenEBS on persistent disks from the cloud providers, converting these more performant and less-expensive ephemeral resources into the foundation for resilient storage, thereby saving money while boosting performance.
As stated before, the mileage may vary from case to case, so feel free to contact us about your unique scenario. We are seeing many ways that necessary trade-offs between performance, resource usage, and workload and storage configurations can be better optimized on a per-workload basis. If you test many permutations of storage configurations against workloads configured in many ways, you can quickly find yourself in test scenarios that could take years to complete (gulp). It is far better to decompose the problem down to workloads so that the problem becomes more tractable. For example, you can configure MySql and the underlying OpenEBS storage differently depending on use case, and even have systems in place that alter the configuration as needed. In other words, a fundamental advantage of the CAS architecture is that it enables a level of customization and refinement that shared everything storage approaches of the past made impossible.
Thanks for reading. Feedback and questions are welcome.
This article was first published on Jan 30, 2019 on OpenEBS's Medium Account
Game changer in Container and Storage Paradigm- MayaData gets acquired by DataCore Software
Don Williams
Don Williams
Managing Ephemeral Storage on Kubernetes with OpenEBS
Kiran Mova
Kiran Mova
Understanding Persistent Volumes and PVCs in Kubernetes & OpenEBS
Murat Karslioglu
Murat Karslioglu